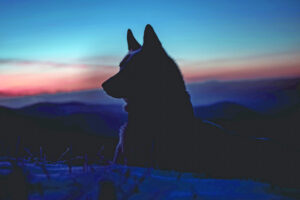
Enriching data with relationship information is expected to turn today’s predominantly narrow AI into a general form closely resembling human insight.
Most AI solutions today are essentially “narrow AI”—focused on performing one task very well, such as facial recognition or chatbots. However, as the technology grows more powerful, it can become “general AI”, which has a much wider range of applications in learning and problem solving.
To make AI more “intelligent” and able to offer more human-like insight and make better decisions, we need to enhance its learning processes, and this requires data.
When people make decisions they nearly always rely on context. If we can feed contextual information to a machine, it can start to replicate human decision-making more closely. Graph data platforms are a key way to add this extra context.
Introducing knowledge graphs
Traditionally, most data science techniques have ignored relationships because it is difficult and slow to manually engineer connected features for machine learning using tabular data.
With graph data platforms, a database is not a simple table of columns and rows but comprises knowledge graphs that include relationships. The data is structured as a series of nodes and connections, storing information about the nature of those connections. All social connections are mapped as nodes and connections.
It is this relationship data that provides the context AI needs to detect patterns and anomalies, and answer more complex questions, such as “What kind of things might be influencing my customers?”
Essentially, a knowledge graph is an insight layer of interconnected data enriched with semantics, so you can reason with the underlying data and use it confidently for complex decision-making.
Four ways to enhance AI learning
There are at least four main areas where graph data platforms provide context and savings for AI.
1. Slashing costs
Knowledge graphs provide context for decision support and ensure that answers are appropriate to the situation, such as directions for autonomous vehicles in rainy driving conditions.
Poorly planned routes can waste time, money and resources. A container shipping company can use knowledge graphs to plan maritime routes. With a knowledge graph, an optimal route based on distances, cost and internal logic can save over 60 kilotons of carbon emissions.
2. Accelerating insights
Graph-accelerated machine learning uses graphs to optimize models and speed up processes. For example, a medical device manufacturer used a knowledge graph to identify at-fault components in its supply chain. The graph enabled it to pinpoint the root causes of problems quickly, reducing query times from two minutes to seconds.
3. Identifying predictive elements
Basing a predictive model on strong characteristics found in the data improves accuracy. This ‘connected-feature extraction’ analyzes data to identify the most predictive elements within data.
One roadside assistance service used a graph database to crowdsource OpenStreetMap data to detect changing roadway and driving conditions, analyze dynamic conditions for developing trends and predict the potential consequences of those trends.
4. Providing transparency
Finally, knowledge graphs offer a way to provide transparency into the way AI makes decisions. This area is called AI “explainability”.
Machine learning and deep learning have been known to provide incorrect answers, such as categorising a dog as a wolf. It can be challenging to understand what led an AI solution to make that decision. A knowledge graph provides the ability to track how data is changed, where data is used and who used what data.
According to Gartner, by 2025, graph technologies will be used in 80% of data and analytics innovations, paving the way for rapid decision making across organisations. As AI technology matures, graphing techniques will become prevalent—going from 13% when AI maturity is lowest, to 48% when maturity is highest.
As organisations look for ways to manage the explosion of data created through digitalisation and IoT, knowledge graphs will be key in making connections and unlocking the insights needed to stay ahead of the competition.
By Alicia Frame, Director, Graph Data Science, Neo4j
This article was first published by Digicon Asia