How can organisations prepare for the rise of generative AI, and what are some best practices for implementing it effectively?
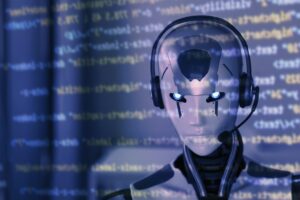
According to the 2023 NewVantage Partners ‘Data and Analytics Leadership Survey, 82.6% of organisations reported having appointed a Chief Data Officer (CDO) or Chief Data and Analytics Officer (CDAO). This is evidence of how seriously companies now take the role and value of data within their organisations. The next stage for businesses is to develop a data strategy that cuts across people, processes, and technology to guide towards achieving business goals and implementing generative AI in an ethical and responsible manner.
Generative AI has the potential to revolutionise businesses in numerous ways. It can automate and optimise complex processes and enhance customer experiences. By using generative AI, businesses can gain valuable insights from large amounts of data, allowing them to make more informed decisions. The most common improvements that generative AI can make in business are increased efficiency and reduced costs.
Additionally, generative AI can provide solutions to problems that require access to significant amounts of research, this can be seen in areas where it is difficult to stay up-to-date on new developments, like biotechnology, clean energy, infectious diseases, and genetic engineering.
So how can businesses get started?
To effectively implement generative AI in business, there are several key steps that should be followed. Firstly, it is important to clearly define the business objectives that generative AI is being used to achieve. This involves identifying the specific problem that needs to be solved, the desired outcome, and the metrics that will be used to measure success.
For example, the issue could be to reduce the number of clicks it takes online customers to search products by keyword, then filter, then sort, then browse results.
Once the objectives have been defined, the next step is to gather high-quality data. Since generative AI heavily relies on data, it is essential to ensure that the data being used is accurate, relevant, and of high quality. This may involve cleaning and pre-processing the data to remove inconsistencies, biases, and errors.
The model needs to be developed and trained using algorithms and machine-learning techniques. This involves selecting the appropriate model architecture, fine-tuning parameters, and validating the model using test data. This may involve integrating the model with existing systems and processes, ensuring that it is secure and scalable, and monitoring its performance over time.
Finally, it is important to continuously evaluate and improve the generative AI model. This involves monitoring the model’s performance, collecting feedback from users, and iterating on the model to improve its accuracy and relevance. This last step is critical, and where we have seen businesses receive backlash – when biases in the data manifest and highlight areas that haven’t been properly reviewed.
How do you see the field of generative AI evolving in the next 5-10 years, and what new developments or applications do you anticipate?
Generative AI has the potential to make significant strides in addressing some of the world’s most pressing issues in the next 5 to 10 years. Climate change requires immediate and sustained action. Generative AI can contribute to this effort by analysing data on climate patterns, weather, and other variables to help predict and prevent natural disasters. Based on world-leading reductions in CO2, generative AI can recommend policy and legislative changes to guide implementable changes that consider geolocation, GDP and cultural factors. By providing more accurate and timely insights into these events, generative AI can help communities and governments prepare for and mitigate the effects of climate change.
Improving aspects of the food industry, including food redistribution, production, and retail operations is another area we expect to see significant growth. In the case of food redistribution, generative AI can help optimise the distribution of surplus food from restaurants, grocery stores, and other food businesses to organisations that support vulnerable populations such as food banks and homeless shelters. By analysing data on food availability, demand, and transportation networks, generative AI algorithms can generate optimised routes and schedules for food redistribution, which can reduce waste and ensure that surplus food is distributed to those who need it most. Generative AI can also optimise food production in urban areas, which can help reduce waste and increase food security.
Generative AI can also be applied to food retail operations in urban areas, which can help reduce waste and improve sustainability. By analysing data on consumer demand, inventory levels, and other variables, generative AI algorithms can generate optimised stocking and ordering schedules for grocery stores and other food retailers. This can help reduce waste by ensuring that perishable goods are sold before they expire, and by reducing overstocking and spoilage. The implementation of generative AI in the food industry has the potential to optimise food distribution, production, and retail operations in urban areas and significantly improve sustainability and access to food.
By Melissa Voderberg, Associate Director, Customer Experience and Innovation Consulting at Publicis Sapient.
This article was first published by Disruptive Tech Asia