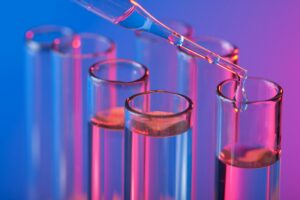
In life sciences, the adoption of new standards such as Study Data Tabulation Model (SDTM) and Analysis Data Model (ADaM) is proving critical for efficient and effective data management and sharing. SDTM provides a new way of organising human clinical and nonclinical study data tabulations, which is required for data submission to regulatory bodies like the United States Food and Drug Administration (FDA) and Pharmaceuticals and Medical Devices Agency (PMDA) Japan, while ADaM defines dataset and metadata standards for clinical trial statistical analyses, ensuring efficient generation, replication, and review of data.
The Clinical Data Interchange Standards Consortium (CDISC) 360 is another important initiative that aims to implement standards as linked metadata to support metadata-driven automation across the entire clinical research data lifecycle, making it easier for researchers to analyse and share their findings.
The digital transformation of pharma industry regulatory processes, hence, has started to make data a key tool. However, with the increasing volume and complexity of data generated in drug discovery and clinical research, life sciences R&D practitioners need better ways of organising, structuring and exploiting their data, at scale.
To address the increasing volume and complexity of data generated in drug discovery and clinical research, the sector is increasingly adopting an innovative data structure approach, the knowledge graph. Graph databases can tackle complex problems in drug discovery, multiomics, and clinical research by allowing researchers to store and analyse complex interconnected data such as relationships between genes, proteins, cells, and tissues, as well as help the sector get better at meeting standards like SDTM and AdaM.
The main advantage knowledge graphs offer is their basic design. Unlike traditional SQL databases that use fixed tables with rows and columns to store data, knowledge graphs represent data as interconnected ‘nodes’ (or entities) linked by ‘edges’ (or relationships).
This network (a graph is a mathematical name for a network) of interconnections holds the key to unlocking breakthrough insights. The power of knowledge graphs is evident in their ability to represent complex data relationships. In the Panama Papers work, for example, a knowledge graph helped uncover an intricate network of opaque offshore accounts, shell companies, and individuals allowing investigators to connect the dots and uncover hidden relationships. These insights would have been difficult to detect using traditional data analysis methods.
Owing to their ability to represent intricate data, knowledge graphs have many applications beyond financial investigations. One such area is biological science, where knowledge graphs can capture the intricate interconnections and correlations among diseases, genes, environment, diet, behaviour, and other factors.
Analysis of such connections and correlations leads to a more profound understanding of the domain, enabling faster and more significant deductions. With the advent of modern native graph databases, cross-comparisons involving billions of connections can be carried out at scale, facilitating the identification of hidden patterns and connections. This ability has the potential to revolutionise biotech and medicine.
AI algorithms applied to patient data
AstraZeneca is leveraging the power of knowledge graphs to facilitate reaction and synthesis prediction, streamlining the development of novel organic molecules and even demonstrating the potential of knowledge graphs in reaction and synthesis prediction during drug discovery. The firm is working with a nine-million-node graph featuring 33 million relationships to do this, with the graph helping identify areas in the chemical space where new reaction networks can be formulated.
According to the firm, graphs are useful in drug discovery because chemical reactions naturally form networks. When a reaction occurs, the product can lead to other reactions, resulting in a graph structure. By utilising path queries between two molecules, data scientists can understand the connections between reactions. This information can help train new lead prediction algorithms, enabling scientists to predict how different molecules will react and improve drug discovery efforts. AstraZeneca’s application of graph technology is being supplemented with data visualisation tools so that scientists can recognise important molecules and reactions they want to investigate more quickly.
AstraZeneca is one of many pharma brands benefiting from knowledge graphs. GSK, for example, finds graph techniques and tools are reducing the manual effort required to validate analysis to ‘nearly zero’ and ensuring compliance with GDPR on informed consent so that the patient’s details disappear from downstream renderings of the data.
In addition to using knowledge graphs to enhance GSK’s clinical reporting workflows and address emerging regulatory standards, GSK aims to proactively perform risk-based monitoring. Here, the GSK team has developed a Google-like question-and-answer system that enables users to obtain rapid answers from their clinical trial data. GSK is also employing powerful AI algorithms originally developed for pre-clinical data sets which can be applied to patient-level clinical data. To manage the dataset effectively, the company has opted for a clinical knowledge graph that provides a patient-centric data model, which integrates all domain silos and enables everyone involved to understand the clinical data. GSK is on the way to achieving this, says the team, and while this project isn’t yet a full industrial process, early results are consistently strong.
Both AstraZeneca and GSK say graph technology was the natural fit for this problem space.
One of the main benefits of knowledge graphs is that they are not restricted by particular data schema or formatting requirements. They can work with native data structures, and queries can be conducted by asking relevant questions. Moreover, these queries can be executed at lightning-fast speeds, often up to 3,000 times faster than SQL database queries, and across dense networks of knowledge. Such speed can enable rapid pinpointing of the best doctor to target for a clinical trial’s success, considering not only their area of expertise but their current capacity, access to the necessary equipment, and whether they may be working with a competitor.
Clinical trials can benefit greatly from knowledge graphs in the case of rare conditions where small patient populations can make it difficult to achieve statistical significance. For example, in diabetes research, knowledge graphs can aid in phenotype mapping, where researchers want to understand the relationship between different observable phenotypes in both humans and animals. This can be particularly challenging when the clinical parameters and observations used to measure these phenotypes are not directly comparable between species.
Another use case that shows more of the benefits of knowledge graphs is Novartis, which uses knowledge graphs to connect and navigate the vast amounts of data it has accumulated over the years. By using graph technology to create a central database of biological data, the firm is starting to be able to link genes, diseases, and compounds in patterns that allow researchers to quickly identify and investigate correlations between them.
To take one example, text mining is used at the beginning of the drug development pipeline to extract relevant text data from PubMed, which is then combined with Novartis’s historical and image data in a knowledge graph. The team then uses graph algorithms to identify desired triangular node patterns, allowing them to find data linked by the desired node pattern and arrange the triangles according to a metric that gauges the associated strength between each node in each triangle.
Knowledge graphs in pharma R&D
Overall, the Novartis R&D team has found that using knowledge graphs has allowed it to navigate its vast amounts of data more flexibly, helping to accelerate drug discovery and develop the next generation of medicines.
Novartis, AstraZeneca and GSK are far from being alone. As society faces increasingly demanding, complex clinical challenges, understanding the value of relationships between data with the help of advanced tools like a graph-based knowledge graph is emerging as important as the data points themselves. Without the ability to mine correlations for new insights, even the most promising innovations may lack context and researchers may struggle to make much headway. Based on these and other life sciences use cases, it’s becoming ever-more evident that, with their ability to uncover insights from complex data sets, knowledge graphs are starting to play an increasingly vital role in pharma R&D.
By Laxman Singh, Neo4j Head, ASEAN and India
This article was first published by BioSpectrum Asia