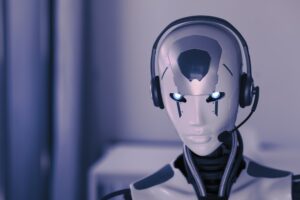
Artificial Intelligence (AI) is showing increased adoption in customer service. Traditionally, the primary role for customer-facing chatbots has been to reduce the volume of inbound requests to human operators. But now, Al-powered assistants are also being deployed behind-the-scenes for contact-centre agents.
When these technologies are able to leverage vast amounts of customer data, they can become extremely powerful. Retailers are recognising the need to be smarter and more personalised in their interactions with customers. Collecting customer information – Know Your Customer – has been a leading trend in recent years.
However in the post-cookie, pro-privacy era, this is becoming increasingly complex. Both retailers and suppliers are faced with the challenge of finding novel ways to connect with customers that are technically feasible and legally compliant.
The advantages of personalisation
Retailers have a unique advantage when it comes to customer data, due to their deep knowledge derived from transactional history. It is still permissible to collect and store his first-party data. Armed with a huge depth of knowledge about an individual customer, from their browsing behaviour to their purchase patterns, both human and AI-powered agents can deliver exceptionally personalised service.
Data analytics and Al can also detect wider patterns in customer data to predict how an individual customer might behave in the future. Even if they haven’t bought a product yet, the behaviour of other customers may indicate that they will. Recommendation engines are believed to drive up to 35 per cent of Amazon’s sales.
Personalisation also drives loyalty. A tailored experience fosters a sense of being valued and understood, that a company knows the consumer and understands their needs. This creates stronger emotional connections with a brand and makes customers more likely to return.
The risks of personalisation
But it’s very easy for this personalisation to cross the line into intrusiveness. An obvious example would be a customer who previously bought intimate products or medical supplies, and might be uncomfortable with a human or bot “remembering” this and referring to it directly in a chat or indirectly through product recommendations and promotions.
It is critical to get the technology right. Examples also abound of chatbots becoming inappropriate, such as expressing racism or even trying to convince one user to divorce his wife.
Another risk is ‘uncanny valley’ territory. This is a term used to describe robots or CGI figures that seem almost human but have a flaw or imperfection that people find creepy. To combat this, chatbots could be programmed to be more factual and less human-like.
The challenge is striking a balance: an overly robotic chatbot might reduce the level of engagement that companies are secking. Earlier generations of Al solutions were often more robotic in nature, following strict logic. This often frustrated customers, who struggled to ask a question in a way the bot could understand and answer. Association for Computing Machinery studies suggest that more human-like chatbots can reduce stress in users.
Conclusion
The key is transparency and choice: allowing customers to opt out if they are uncomfortable. While it’s important to deliver the right message to the right customers at the right time, and to facilitate this for suppliers as well, not all customers want to be messaged. In a pool of 18 million rewarded customers, only 6 million may be open to communication.
Discouraging customers from opting out requires a delicate equilibrium between leveraging Al and personalisation, and overwhelming them. The goal is to find the Goldilocks zone’ – communications that are neither too frequent and invasive nor too infrequent and invisible.
Generative AI (GenAI) is well suited for customer service, but the responses need to be factual and if there is uncertainty, that should be communicated to the customer. A good data scientist can tune a GenAl model to be more factual and allow for uncertainty.
In today’s interconnected world, organisations can no longer afford to operate in isolation. Integration and communication across channels are essential. It’s vital for the right hand to know what the left hand is doing to ensure a cohesive and effective customer engagement strategy. In the current era of advanced GenAl and personalised experiences, a careful balance must be struck to avoid crossing into the realm of creepiness.
By John Costello, chief technology officer of Publicis Sapient
This article was first published by Inside Retail